主持人:周涛 副教授
时间:2023年5月8日(周一)上午10:00
地点:犀浦校区3教30345会议室
题目:The Expressivity of Quantum Neural Networks: Unitary and Beyond
报告人: 吴亚东 复旦大学博士后
简介: 吴亚东,复旦大学博士后。2016年本科毕业于中国人民大学。2016-2021年于清华大学高等研究院攻读博士学位。主要从事机器学习算法及其在量子物理中的应用,量子机器学习与量子信息的相关研究。
摘要:Machine learning and quantum computing are both two hop topics. Machine learning has got great success in data analysis, auto-driving, and so on. While quantum computing has quantum supremacy beyond the classical computing and many scientists are devoted to realize the universal quantum computing. However, quantum mechanics preserves the quantum system or the physical observable evolved unitarily, which means it’s impossible to learn accurate information about the entire system from the single qubit observable. In this talk, I focus on the quantum neural network (QNN), one machine learning model in the quantum system. We discuss the expressive ability of QNNs in three aspects. Firstly, for the unitary QNN, we propose an averaged operator size to describe the information scrambling ability of a given QNN architecture. We found that for the architecture with a larger averaged operator size, the learning efficiency is higher during training. This gives us a guiding principle to design a QNN architecture with high efficiency. Secondly, we find that with the ancillary qubit and(or) replicas, which would enlarge the Hilbert space, QNNs will learn linear observables like magnetization and non-linear observables like entanglement entropy with high accuracy. Thirdly, going beyond the unitary constraints, we add the random unitary ensemble in QNNs. The random unitary ensemble will extract the information beyond the unitary evolution and enhance the expressive ability of QNNs.
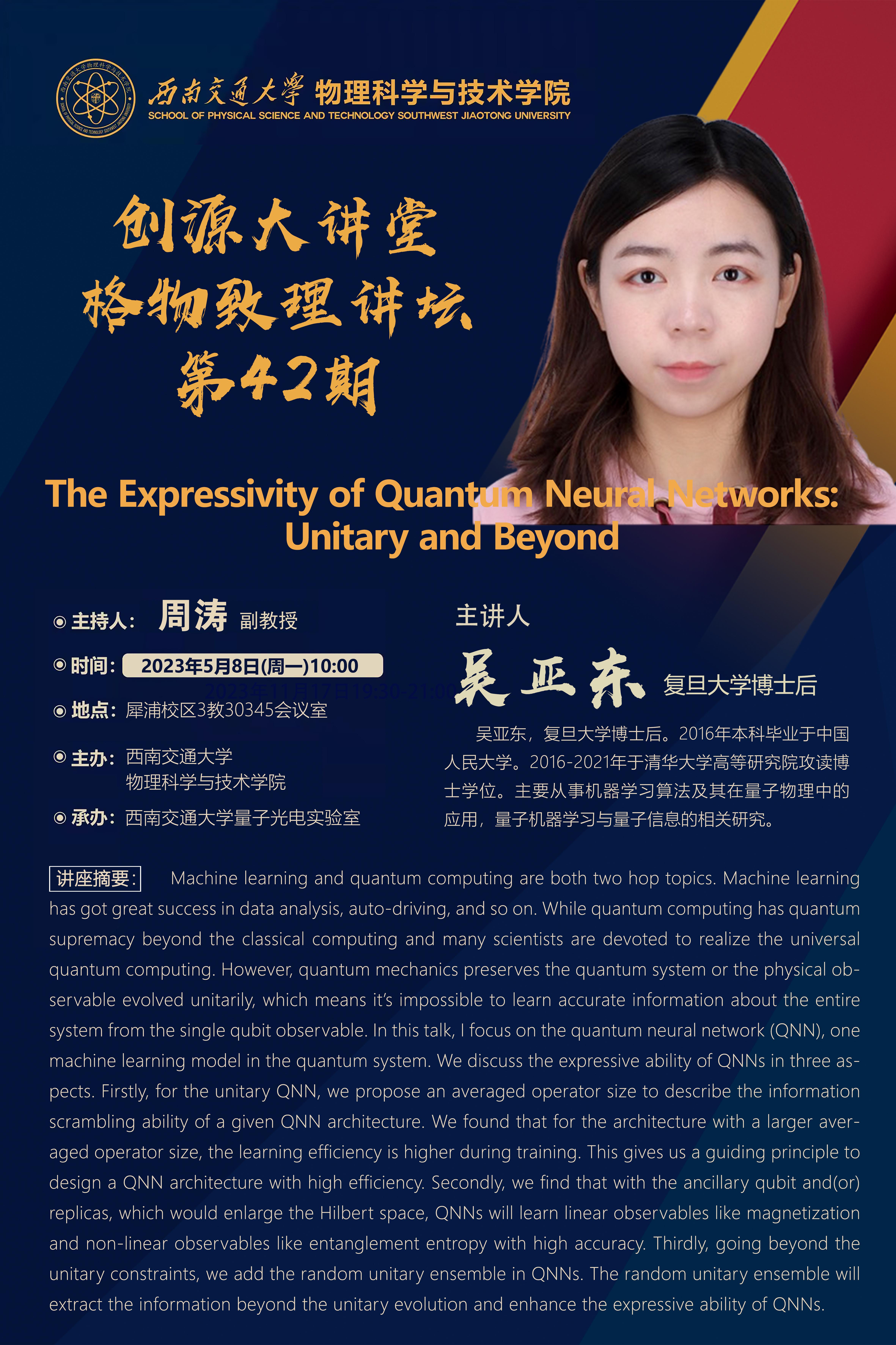